Using Machine Learning to Predict Problematic Prescription Opioid Use and Opioid Overdose
PI: Walid Gellad, MD, MPH
Funding Source: NIH/NIDA
September 2017 - June 2022
This R01 project improves on traditional methods for predicting risk of overdose from prescription opioids by applying machine learning techniques. The study team, led by Dr. Gellad, will develop prediction algorithms to identify patients who are at risk of opioid overdose, and after testing and refining the algorithm, will compare the accuracy of an approach integrating Medicaid claims data with clinical data to an approach using claims data alone.
Read about this work in the media
Machine-learning Algorithm Predicts Future Mortality Following Non-fatal Opioid Overdose
Read published results from this work

From: Evaluation of Machine-Learning Algorithms for Predicting Opioid Overdose Risk Among Medicare Beneficiaries With Opioid Prescriptions, JAMA Network Open, March 22, 2019

From: Using machine learning to predict risk of incident opioid use disorder among fee-for-service Medicare beneficiaries: A prognostic study, PLOS One, July 17, 2020

From: Occupational Patterns of Opioid-Related Overdose Deaths Among Arizona Medicaid Enrollees, 2008-2017, JGIM, July 2020
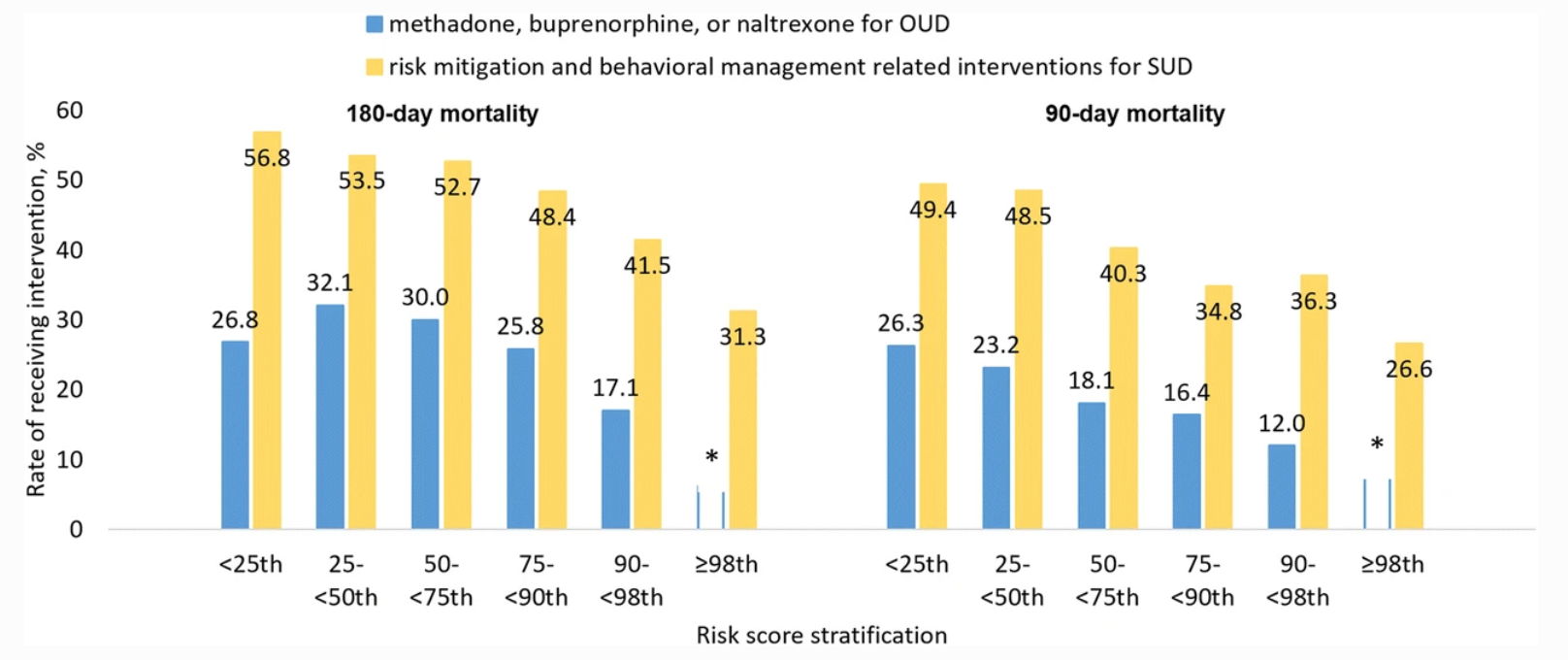
From: Predicting Mortality Risk After a Hospital or Emergency Department Visit for Nonfatal Opioid Overdose, JGIM, January 2021
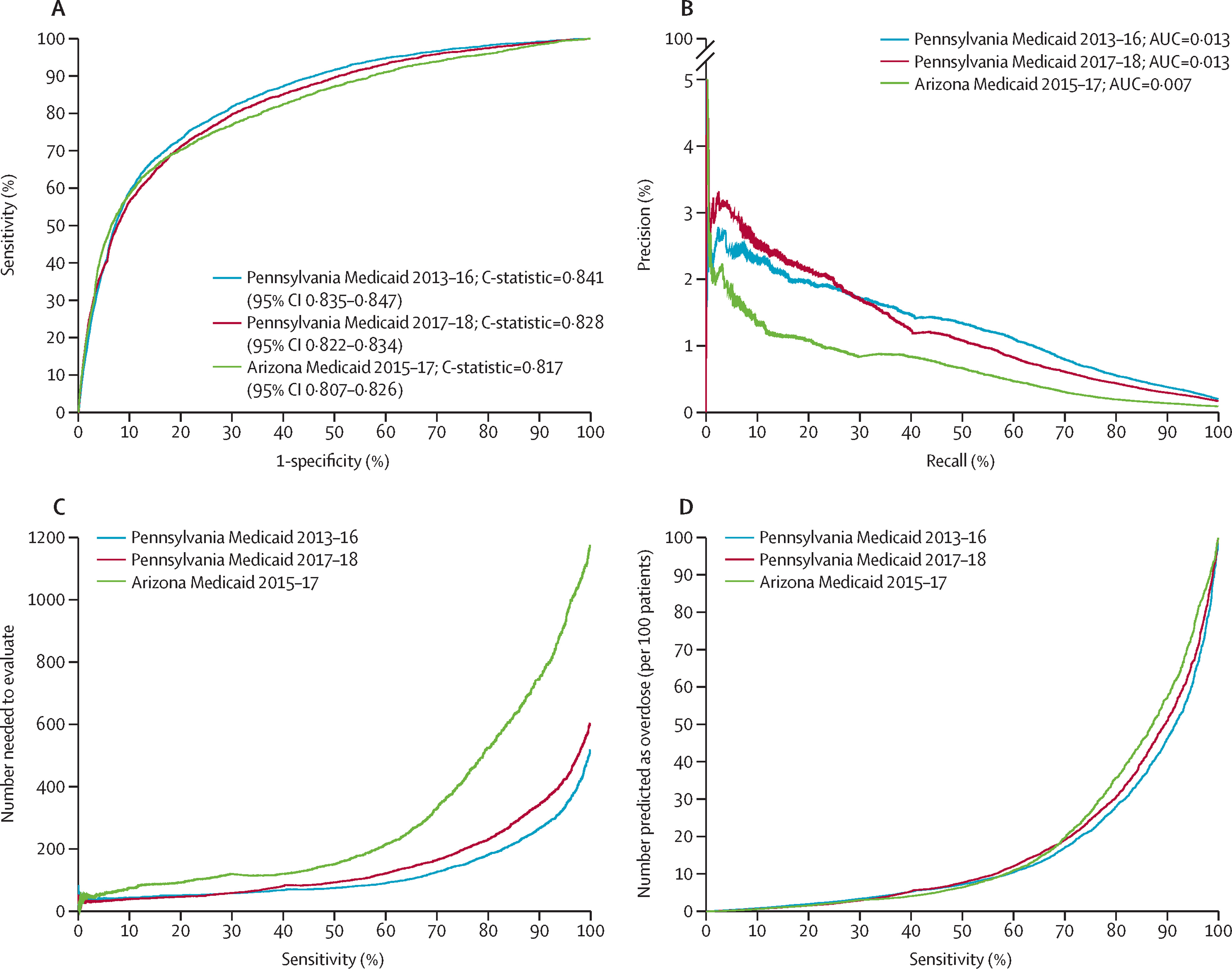
From: Developing and validating a machine-learning algorithm to predict opioid overdose in Medicaid beneficiaries in two US states: a prognostic modelling study, The Lancet Digital Health, May 2022
Machine-learning Algorithm Predicts Future Mortality Following Non-fatal Opioid Overdose
Read published results from this work

From: Evaluation of Machine-Learning Algorithms for Predicting Opioid Overdose Risk Among Medicare Beneficiaries With Opioid Prescriptions, JAMA Network Open, March 22, 2019

From: Using machine learning to predict risk of incident opioid use disorder among fee-for-service Medicare beneficiaries: A prognostic study, PLOS One, July 17, 2020

From: Occupational Patterns of Opioid-Related Overdose Deaths Among Arizona Medicaid Enrollees, 2008-2017, JGIM, July 2020
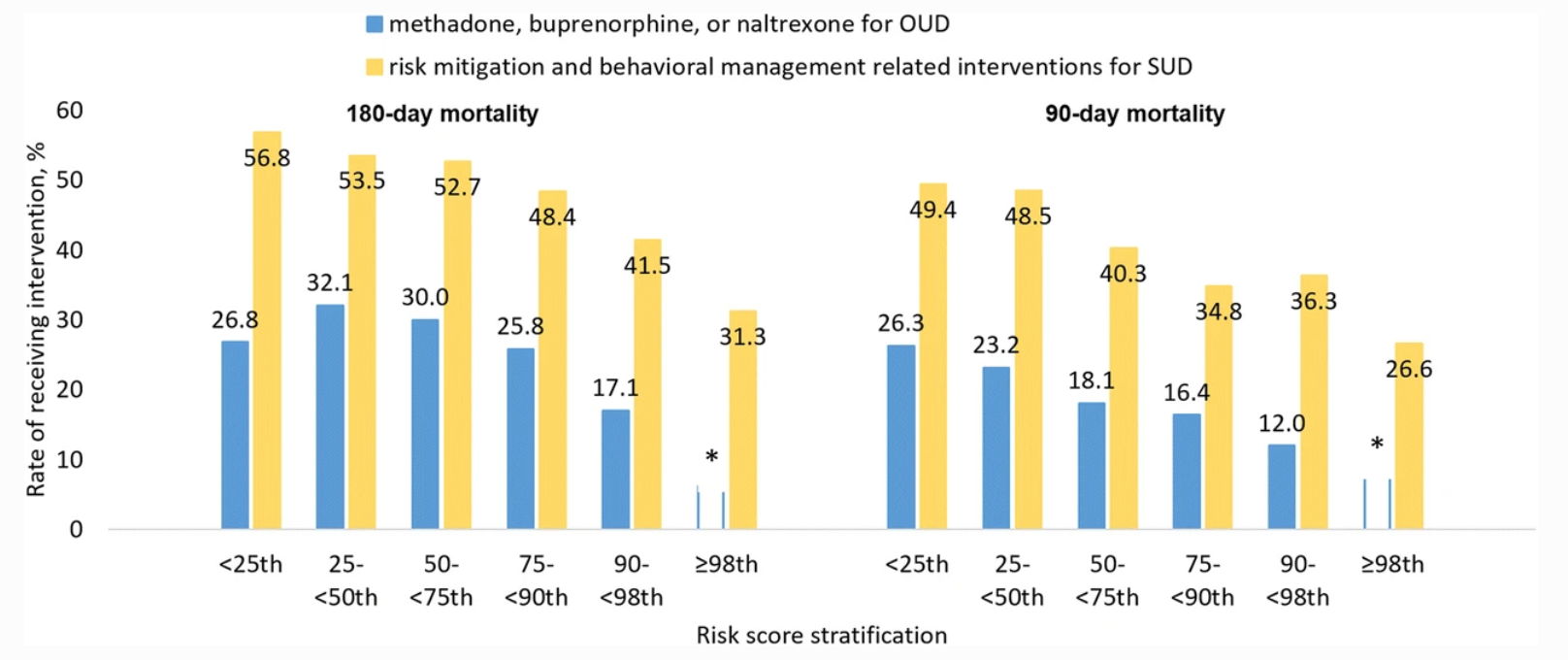
From: Predicting Mortality Risk After a Hospital or Emergency Department Visit for Nonfatal Opioid Overdose, JGIM, January 2021
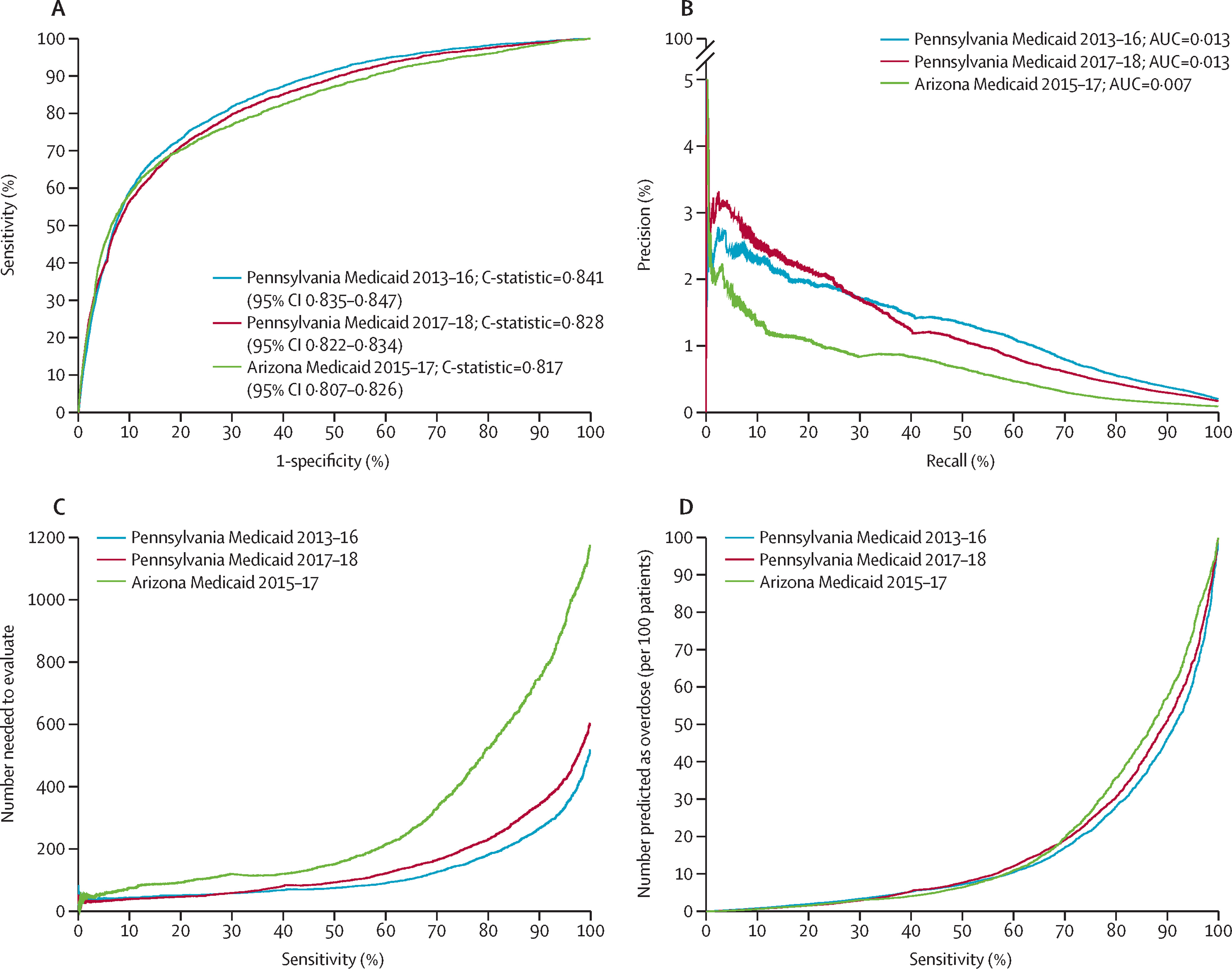
From: Developing and validating a machine-learning algorithm to predict opioid overdose in Medicaid beneficiaries in two US states: a prognostic modelling study, The Lancet Digital Health, May 2022